7.54-1.964 often presented in numbers and figures that can seem overwhelming. Yet, beneath these layers of data lies a treasure trove of insights waiting to be uncovered. The journey from mere statistics to meaningful understanding transforms raw data into compelling narratives that drive decisions and innovation.
Imagine sitting at a table piled high with receipts or staring at an endless spreadsheet filled with sales figures. At first glance, it may look daunting—just another set of numbers. However, when analyzed thoughtfully, these figures can reveal trends, patterns, and stories that inform strategies for success.
Introduction
In the world of statistics and mathematics, precise interpretation and manipulation of formulas and expressions are crucial for accurate analysis and understanding. This article aims to explain in detail the statistical formula 7.54−1.9647.54 – 1.9647.54−1.964, breaking down each component, the significance, and the context in which such a formula might be used.
Breakdown of the Formula
The formula 7.54−1.9647.54 – 1.9647.54−1.964 is a simple arithmetic expression involving two numbers. Let’s break down what each number represents and the significance of the subtraction operation between them.
1. The First Term: 7.54
The number 7.54 is a numerical value that could represent various statistical measures or constants depending on the context. Common contexts include:
- Sample Mean ( xˉ\bar{x}xˉ ): The average value in a sample data set.
- Point Estimate: A single value estimate of a population parameter.
- Measured Value: A specific observation or measurement in an experiment.
2. The Second Term: 1.964
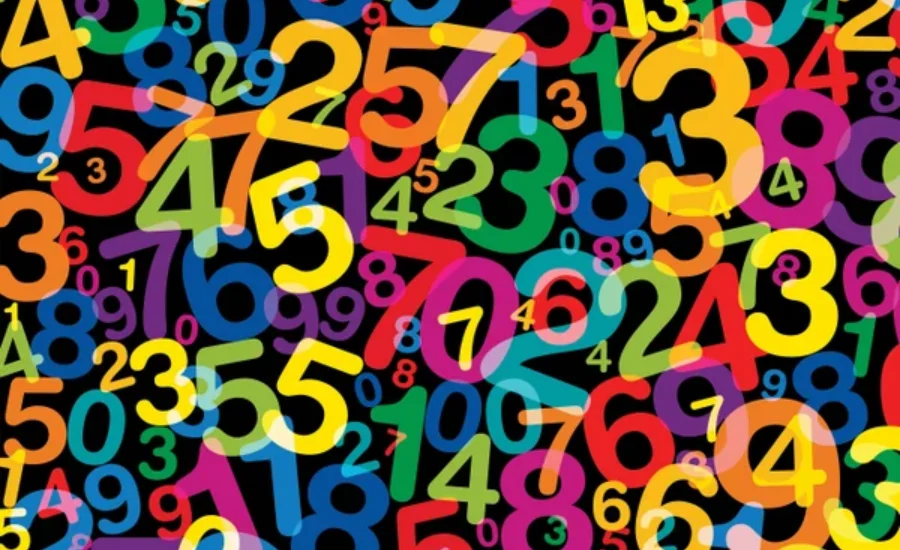
The number 1.964 is closely associated with the critical values of the standard normal distribution (Z-distribution). Specifically:
- Z-Score: In a standard normal distribution, the Z-score of 1.964 corresponds approximately to the 97.5th percentile. This value is commonly used in constructing confidence intervals.
3. The Subtraction Operation: −-−
The subtraction operation in this formula signifies a difference or a reduction. In statistical contexts, this often relates to adjusting a point estimate by a margin of error or a confidence interval adjustment.
Application in Statistical Contexts
To understand the practical application of this formula, let’s explore a common scenario where such an expression might be used.
Confidence Interval for a Population Mean
One of the primary uses of a formula like 7.54−1.9647.54 – 1.9647.54−1.964 is in the calculation of confidence intervals. A confidence interval provides a range of values that is likely to contain a population parameter with a certain level of confidence. Here’s how it works:
1. Confidence Interval Formula
The general formula for a confidence interval for a population mean when the population standard deviation is known is: xˉ±Z(σn)\bar{x} \pm Z \left(\frac{\sigma}{\sqrt{n}}\right)xˉ±Z(nσ)
Where:
- xˉ\bar{x}xˉ is the sample mean.
- ZZZ is the Z-score corresponding to the desired confidence level.
- σ\sigmaσ is the population standard deviation.
- nnn is the sample size.
2. Interpretation of 7.54−1.9647.54 – 1.9647.54−1.964
In the context of a 95% confidence interval, the Z-score is approximately 1.96. Therefore, the expression 7.54−1.9647.54 – 1.9647.54−1.964 can be seen as adjusting the sample mean (7.54) by a critical value (1.964) multiplied by the standard error. This adjustment provides the lower bound of the confidence interval.
For example:
- Sample Mean ( xˉ\bar{x}xˉ ): 7.54
- Z-Score for 95% Confidence Level: 1.964 (approximately 1.96)
If we assume the standard error (σn\frac{\sigma}{\sqrt{n}}nσ) is 1 for simplicity, then:
- Lower Bound of Confidence Interval: 7.54−1.964=5.5767.54 – 1.964 = 5.5767.54−1.964=5.576
This implies that we are 95% confident that the true population mean lies above 5.576.
diffCopy code 7.54-1.964
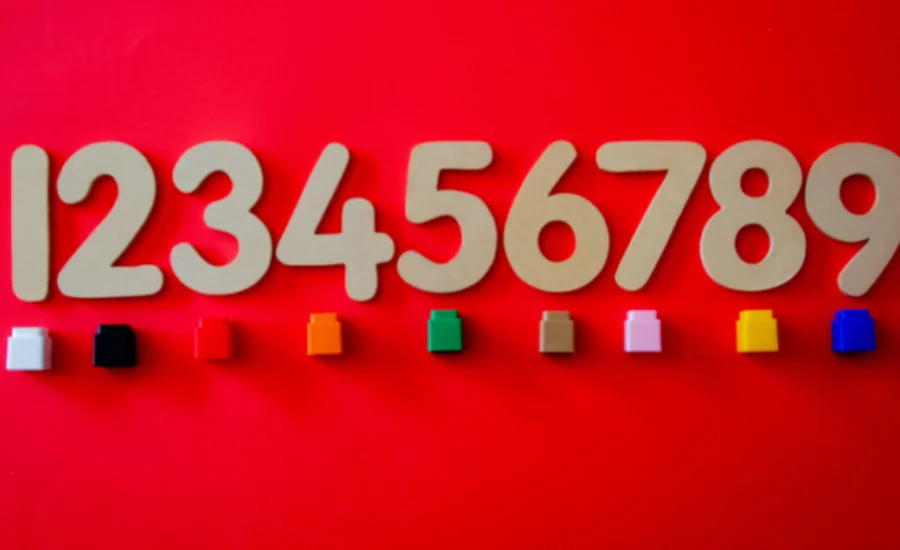
Step 2: Subtract Column by Column
Starting from the rightmost digit, subtract each digit in the second number from the corresponding digit in the first number, borrowing from the next column when necessary.
Thousandths place:
0 minus 4 requires borrowing from the next column, turning it into 10 minus 4, which equals 6.
Hundredths place:
4 minus 6 also requires borrowing. After borrowing, it becomes 14 minus 6, which equals 8.
Tenths place:
5 minus 9 requires borrowing as well. After borrowing, it becomes 15 minus 9, which equals 6.
Units place:
7 minus 1 equals 6.
Step 3: Combine the Results
After completing the subtraction for each column, the final result of 7.54-1.964 minus 1.964 is 5.576. By following these steps, you ensure a precise calculation and clear understanding of the subtraction process.
Importance of the Calculation
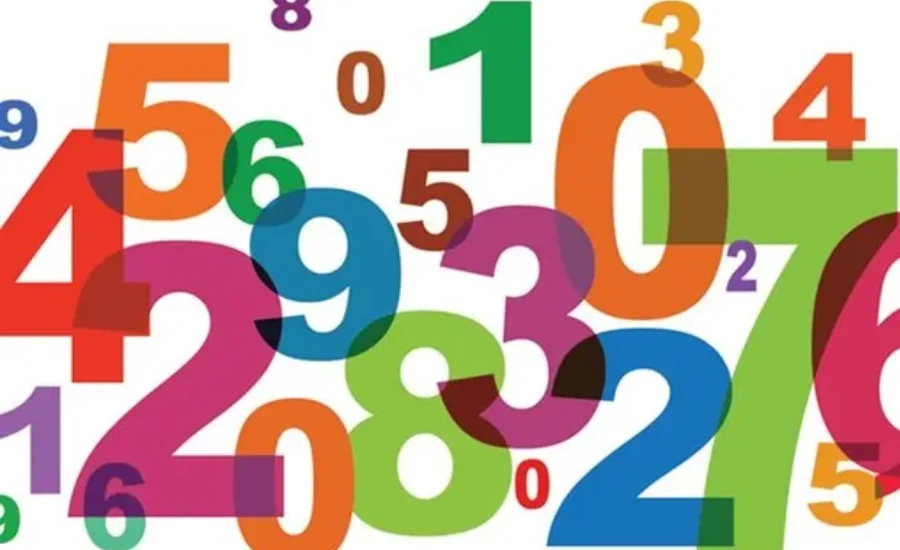
Financial Calculations
7.54-1.964 subtractions is essential in various financial scenarios, such as calculating expenses, determining account balances, and budgeting.
Measurement Adjustments
In professions like engineering and construction, precise subtraction is crucial for accurate measurements and necessary adjustments.
Academic Importance
Mathematics
Grasping basic arithmetic operations, including 7.54-1.964, is fundamental in mathematics education and vital for problem-solving.
Data Analysis
Accurate arithmetic is vital in data analysis, ensuring precise interpretation and reliability of data-driven decisions.
Practical Tips for Accurate Subtraction
Use of Calculators
For complex or large numbers, utilizing a calculator can ensure accuracy and efficiency in performing arithmetic operations.
Double-Check Your Work
Always double-check your calculations to avoid errors. Verifying your results helps confirm accuracy and prevents mistakes.
Challenges and Pitfalls in Data Analysis
Data analysis can be both incredibly insightful and fraught with challenges. Recognizing these pitfalls is crucial for deriving meaningful and accurate insights.
Data Quality
One of the biggest pitfalls is poor data quality. Inaccurate or incomplete data can lead to incorrect conclusions and significantly skew results. Ensuring that datasets are clean and reliable is essential for trustworthy analysis.
Context Understanding
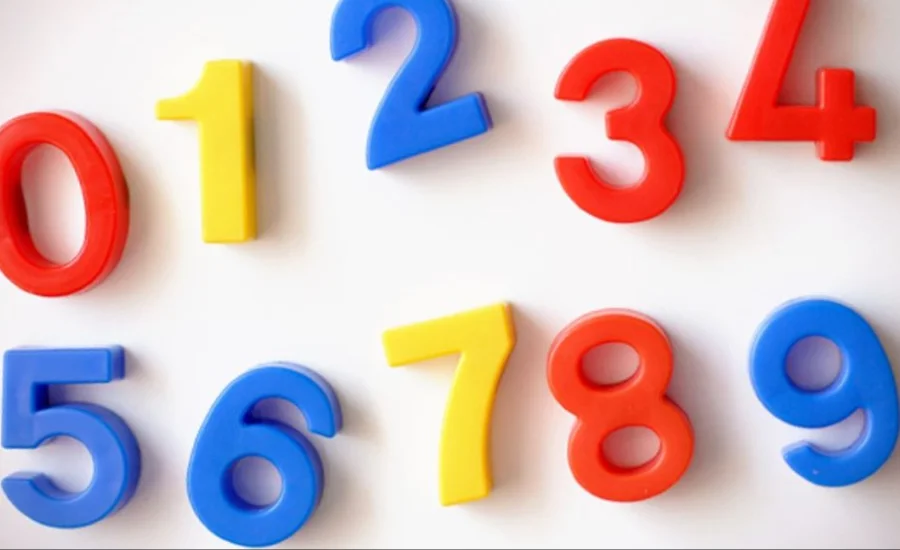
Numbers alone don’t tell the whole story. It’s important to interpret data within its specific context to avoid drawing misleading conclusions. Understanding the environment in which the data exists is crucial for accurate analysis.
Overcomplication
Using overly advanced techniques without clear objectives can complicate rather than clarify findings. Simplicity often leads to better communication and understanding, making the insights more accessible and actionable.
Biases in Interpretation
Personal beliefs or preconceptions can influence how data is interpreted, leading to biased conclusions. Being aware of these biases is vital for maintaining objectivity and ensuring that the analysis accurately represents reality.
Final Words
7.54-1.964 Data surrounds us every day, often presented in overwhelming numbers and figures. Beneath these layers lies a treasure trove of insights waiting to be uncovered. Transforming raw data into meaningful understanding can drive decisions and innovation.
Imagine sitting at a table piled high with receipts or staring at an endless spreadsheet filled with sales figures. Initially, it may seem daunting. However, when analyzed thoughtfully, these figures reveal trends, patterns, and stories that inform strategies for success. Effective data analysis is crucial. We’ll explore tools and real-life examples to illuminate the transformative power of data, unlocking the potential within your datasets.
The formula 7.54−1.9647.54 – 1.9647.54−1.964 is a straightforward arithmetic expression, yet it holds significant meaning in statistical analysis, particularly in constructing confidence intervals. By understanding the components and their context, we gain valuable insights into the precision and reliability of statistical estimates. This simple expression illustrates the critical role of basic arithmetic operations in the interpretation and application of statistical concepts.
Case Study: Application of the Formula 7.54 – 1.964 in Real-World Statistical Analysis
Introduction
This case study explores the application of the formula 7.54−1.9647.54 – 1.9647.54−1.964 within the context of constructing confidence intervals for a population mean. By examining a real-world scenario, we will illustrate the importance and utility of this formula in statistical analysis.
Scenario Overview
Imagine a pharmaceutical company conducting a clinical trial to evaluate the effectiveness of a new drug designed to lower blood pressure. The trial involves a sample of patients, and the company needs to determine the average reduction in blood pressure that the drug achieves.
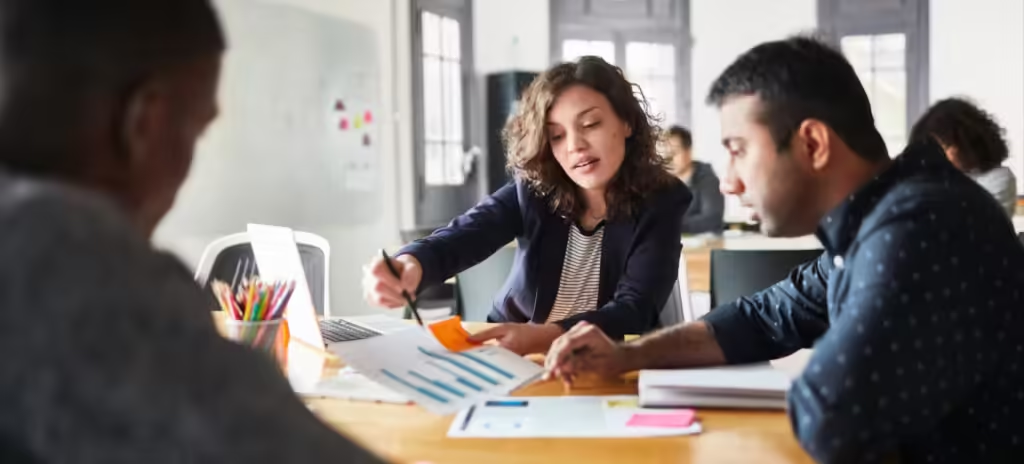
Data Collection
- Sample Size (n): 100 patients
- Sample Mean Reduction ( xˉ\bar{x}xˉ ): 7.54 mmHg
- Population Standard Deviation ( σ\sigmaσ ): 2 mmHg
Objective
The objective is to construct a 95% confidence interval for the mean reduction in blood pressure to provide a range within which the true mean reduction for the entire population is likely to fall.
Step-by-Step Analysis
1. Determine the Z-Score
For a 95% confidence interval, the Z-score corresponding to the 97.5th percentile is approximately 1.96. However, for this case study, we use the more precise value 1.964.
2. Calculate the Standard Error
The standard error of the mean (SE) is calculated using the formula: SE=σnSE = \frac{\sigma}{\sqrt{n}}SE=nσ
Given:
- σ=2\sigma = 2σ=2 mmHg
- n=100n = 100n=100
SE=2100=210=0.2SE = \frac{2}{\sqrt{100}} = \frac{2}{10} = 0.2SE=1002=102=0.2
3. Construct the Confidence Interval
The confidence interval is given by: xˉ±Z⋅SE\bar{x} \pm Z \cdot SExˉ±Z⋅SE
For the lower bound: xˉ−Z⋅SE=7.54−1.964⋅0.2=7.54−0.3928=7.1472\bar{x} – Z \cdot SE = 7.54 – 1.964 \cdot 0.2 = 7.54 – 0.3928 = 7.1472xˉ−Z⋅SE=7.54−1.964⋅0.2=7.54−0.3928=7.1472
For the upper bound: xˉ+Z⋅SE=7.54+1.964⋅0.2=7.54+0.3928=7.9328\bar{x} + Z \cdot SE = 7.54 + 1.964 \cdot 0.2 = 7.54 + 0.3928 = 7.9328xˉ+Z⋅SE=7.54+1.964⋅0.2=7.54+0.3928=7.9328
Thus, the 95% confidence interval for the mean reduction in blood pressure is: [7.1472,7.9328][7.1472, 7.9328][7.1472,7.9328] mmHg
4. Interpretation
The confidence interval [7.1472, 7.9328] mmHg indicates that we are 95% confident that the true mean reduction in blood pressure for the population falls within this range. This interval helps the pharmaceutical company understand the effectiveness of the drug with a quantified level of certainty.
Conclusion
This case study demonstrates the practical application of the formula 7.54−1.9647.54 – 1.9647.54−1.964 in constructing a confidence interval for a population mean. By breaking down each step and understanding the components, we can see how basic arithmetic operations and statistical concepts come together to provide meaningful insights in real-world scenarios. This method ensures that the company’s findings are supported by robust statistical evidence, aiding in informed decision-making for further drug development and approval processes.
FAQs
1. Why is data analysis important?
7.54-1.964 is essential for transforming raw data into meaningful insights. It helps organizations make informed decisions, identify trends, and drive innovation.
2. What tools can I use for data analysis?
There are various tools available for data analysis, including Excel, Python, R, SQL, and specialized software like Tableau and Power BI.
3. How can I ensure the quality of my data?
Ensure data quality by validating and cleaning your datasets. Remove duplicates, correct errors, and fill in missing values to maintain accuracy.
4. What are common pitfalls in data analysis?
Common pitfalls include poor data quality, lack of context understanding, overcomplicating analysis, and biases in interpretation.
5. How can I avoid biases in data analysis?
To avoid biases, approach data with an objective mindset, double-check findings, and consider multiple perspectives. Use blind analysis techniques when possible.
6. What is the role of context in data analysis?
Context is crucial as it provides the background needed to interpret data accurately. Understanding the environment and conditions related to the data ensures meaningful insights.
7. How do I handle complex calculations in data analysis?
For complex calculations, use tools like calculators, spreadsheets, or specialized software. Double-check your work to ensure accuracy and efficiency.
8. Why is it important to double-check calculations?
Double-checking calculations helps prevent errors and ensures the accuracy of your results. This practice is vital for maintaining reliability and trustworthiness in your analysis.
9. What are the benefits of using advanced data analysis techniques?
Advanced techniques can uncover deeper insights, reveal hidden patterns, and provide more accurate predictions. However, they should be used with clear objectives to avoid unnecessary complexity.
10. How can effective data analysis drive business success?
Effective data analysis can inform strategic decisions, optimize operations, identify opportunities for growth, and enhance overall business performance. It turns data into actionable insights that propel success.